10 Ways AI & Machine Learning are Revolutionizing Omnichannel
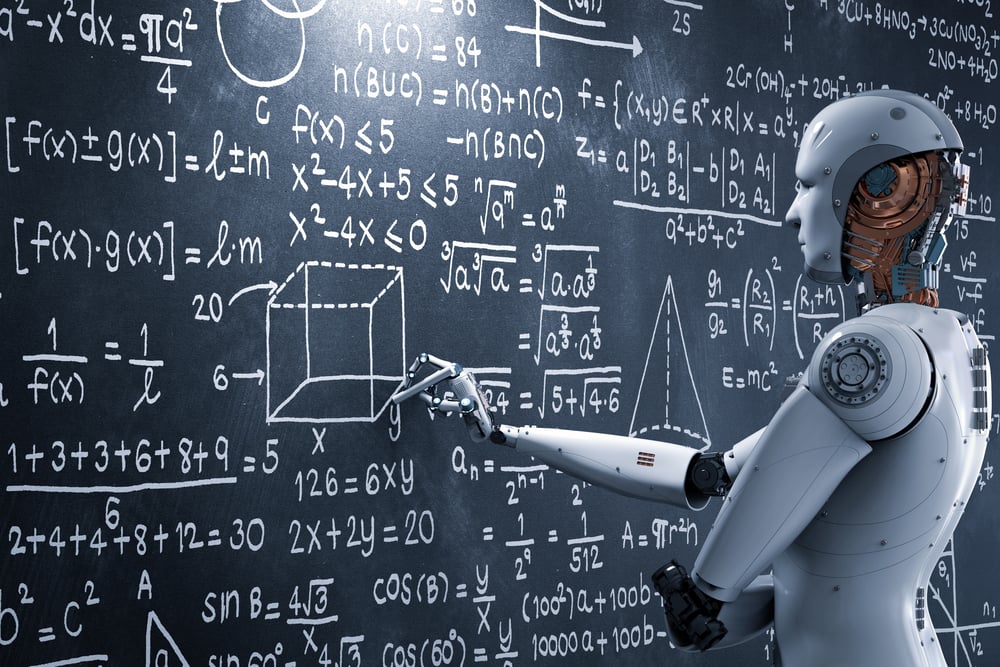
Bottom Line: AI and machine learning are enabling omnichannel strategies to scale by providing insights into the changing needs and preferences of customers, creating customer journeys that scale, delivering consistent experiences.
For any omnichannel strategy to succeed, each customer touchpoint needs to be orchestrated as part of an overarching customer journey. That’s the only way to reduce and eventually eliminate customers’ perceptions of using one channel versus another. What makes omnichannel so challenging to excel at is the need to scale a variety of customer journeys in real-time as customers are also changing.
89% of customers used at least one digital channel to interact with their favorite brands and just 13% found the digital-physical experiences well aligned according to Accenture’s omnichannel study. AI and machine learning are being used to close these gaps with greater intelligence and knowledge. Omnichannel strategists are fine-tuning customer personas, measuring how customer journeys change over time, and more precisely define service strategies using AI and machine learning. Disney, Oasis, REI, Starbucks, Virgin Atlantic, and others excel at delivering omnichannel experiences using AI and machine learning for example.
Omnichannel leaders including Amazon use AI and machine learning to anticipate which customer personas prefer to speak with a live agent versus using self-service for example. McKinsey also found omnichannel customer care expectations fall into the three categories of speed and flexibility, reliability and transparency, and interaction and care. Omnichannel customer journeys designed deliver on each of these three categories excel and scale between automated systems and live agents as the following example from the McKinsey article, How to capture what the customer wants illustrate:
MCKINSEY, HOW TO CAPTURE WHAT THE CUSTOMER WANTS, FEBRUARY, 2019
The foundation all great omnichannel strategies are based on precise customer personas, insight into how they are changing, and how supply chains and IT need to flex and change too. AI and machine learning are revolutionizing omnichannel on these three core dimensions with greater insight and contextual intelligence than ever before.
10 Ways AI & Machine Learning Are Revolutionizing Omnichannel
The following are 10 ways AI & machine learning are revolutionizing omnichannel strategies starting with customer personas, their expectations, and how customer care, IT infrastructure and supply chains need to stay responsive to grow.
1. AI and machine learning are enabling brands, retailers and manufacturers to more precisely define customer personas, their buying preferences, and journeys. Leading omnichannel retailers are successfully using AI and machine learning today to personalize customer experiences to the persona level. They’re combining brand, event and product preferences, location data, content viewed, transaction histories and most of all, channel and communication preferences to create precise personas of each of their key customer segments.
2. Achieving price optimization by persona is now possible using AI and machine learning, factoring in brand and channel preferences, previous purchase history, and price sensitivity. Brands, retailers, and manufacturers are saying that cloud-based price optimization and management apps are easier to use and more powerful based on rapid advances in AI and machine learning algorithms than ever before. The combination of easier to use, more powerful apps and the need to better manage and optimize omnichannel pricing is fueling rapid innovation in this area. The following example is from Microsoft Azure’s Interactive Pricing Analytics Pre-Configured Solution (PCS). Source: Azure Cortana Interactive Pricing Analytics Pre-Configured Solution.
SOURCE: AZURE CORTANA INTERACTIVE PRICING ANALYTICS PRE-CONFIGURED SOLUTION.
3. Capitalizing on insights gained from AI and machine learning, omnichannel leaders are redesigning IT infrastructure and integration so they can scale customer experiences. Succeeding with omnichannel takes an IT infrastructure capable of flexing quickly in response to change in customers’ preferences while providing scale to grow. Every area of a brand, retailer or manufacturer’s supply chain from their supplier onboarding, quality management and strategic sourcing to yard management, dock scheduling, manufacturing, and fulfillment need to be orchestrated around customers. Leaders include C3 Solutions who offers a web-based Yard Management System (YMS) and Dock Scheduling System that can integrate with ERP, Supply Chain Management (SCM), Warehouse Management Systems (WMS) and many others via APIs. The following graphic illustrates how omnichannel leaders orchestrate IT infrastructure to achieve greater growth. Source: Cognizant, The 2020 Customer Experience.
SOURCE: COGNIZANT, THE 2020 CUSTOMER EXPERIENCE.
4. Omnichannel leaders are relying on AI and machine learning to digitize their supply chains, enabling on-time performance, fueling faster revenue growth. For any omnichannel strategy to succeed, supply chains need to be designed to excel at time-to-market and time-to-customer performance at scale. 54% of retailers pursuing omnichannel strategies say that their main goal in digitizing their supply chains was to deliver greater customer experiences. 45% say faster speed to market is their primary goal in digitizing their supply chain by adding in AI and machine learning-driven intelligence. Source: Digitize Today To Future-Proof Tomorrow (PDF, 16 pp., opt-in).
SOURCE: DIGITIZE TODAY TO FUTURE-PROOF TOMORROW (PDF, 16 PP., OPT-IN).
5. AI and machine learning algorithms are making it possible to create propensity models by persona, and they are invaluable for predicting which customers will act on a bundling or pricing offer. By definition propensity models rely on predictive analytics including machine learning to predict the probability a given customer will act on a bundling or pricing offer, e-mail campaign or other call-to-action leading to a purchase, upsell or cross-sell. Propensity models have proven to be very effective at increasing customer retention and reducing churn. Every business excelling at omnichannel today rely on propensity models to better predict how customers’ preferences and past behavior will lead to future purchases. The following is a dashboard that shows how propensity models work. Source: customer propensities dashboard is from TIBCO.

6. Combining machine learning-based pattern matching with a product-based recommendation engine is leading to the development of mobile-based apps where shoppers can virtually try on garments they’re interested in buying. Machine learning excels at pattern recognition, and AI is well-suited for creating recommendation engines, which are together leading to a new generation of shopping apps where customers can virtually try on any garment. The app learns what shoppers most prefer and also evaluates image quality in real-time, and then recommends either purchase online or in a store. Source: Capgemini, Building The Retail Superstar: How unleashing AI across functions offers a multi-billion dollar opportunity.
SOURCE: CAPGEMINI, BUILDING THE RETAIL SUPERSTAR: HOW UNLEASHING AI ACROSS FUNCTIONS OFFERS A MULTI-BILLION DOLLAR OPPORTUNITY.
7. 56% of brands and retailers say that order track-and-traceability strengthened with AI and machine learning is essential to delivering excellent customer experiences. Order tracking across each channel combined with predictions of allocation and out-of-stock conditions using AI and machine learning is reducing operating risks today. AI-driven track-and-trace is invaluable in finding where there are process inefficiencies that slow down time-to-market and time-to-customer. Source: Digitize Today To Future-Proof Tomorrow (PDF, 16 pp., opt-in).
8. Gartner predicts that by 2025, customer service organizations who embed AI in their customer engagement center platforms will increase operational efficiencies by 25%, revolutionizing customer care in the process. Customer service is often where omnichannel strategies fail due to lack of real-time contextual data and insight. There’s an abundance of use cases in customer service where AI and machine learning can improve overall omnichannel performance. Amazon has taken the lead on using AI and machine learning to decide when a given customer persona needs to speak with a live agent. Comparable strategies can also be created for improving Intelligent Agents, Virtual Personal Assistants, Chatbot and Natural Language (NLP) performance. There’s also the opportunity to improve knowledge management, content discovery and improve field service routing and support.
9. AI and machine learning are improving marketing and selling effectiveness by being able to track purchase decisions back to campaigns by channel and understand why specific personas purchased while others didn’t. Marketing is already analytically driven, and with the rapid advances in AI and machine learning, markets will for the first time be able to isolate why and where their omnichannel strategies are succeeding or failing. By using machine learning to qualify the further customer and prospect lists using relevant data from the web, predictive models including machine learning can better predict ideal customer profiles. Each omnichannel sales lead’s predictive score becomes a better predictor of potential new sales, helping sales prioritize time, sales efforts and selling strategies.
10. Predictive content analytics powered by AI and machine learning are improving sales close rates by predicting which content will lead a customer to buy. Analyzing previous prospect and buyer behavior by persona using machine learning provides insights into which content needs to be personalized and presented when to get a sale. Predictive content analytics is proving to be very effective in B2B selling scenarios, and are scaling into consumer products as well.